The AI-first winning formula
To sharpen their competitive edge and foster long-term success, organizations must regard data as a high-value business asset and a basis for developing AI tools.
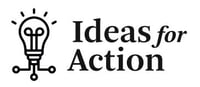
Who are your AI champions?
Who is responsible for data collection, storage and management in your organization? Who will conduct inventories and evaluate data derived from external partners, to ensure it meets agreed standards and is systematically labeled and organized? If no one among your current employees is skilled in data administration then upskilling, task outsourcing or recruitment of new talent are of top priority. Equally important is securing the right people to play the role of “data and AI champions” across various business units to ensure organization-wide adoption and alignment of responsible data management practices.
How AI-ready is your business?
Experts at Roland Berger have established the AI Readiness Radar, a systematic assessment of all AI-related capabilities of a company. It helps businesses to define evaluation parameters to be explored as valuable input for outlining a successful AI-first business strategy.
Get in touch with us to find out more
Money talks louder than chatbots
What if evaluating AI products wasn’t just about accuracy and performance, but also project unit economics at scale? If no clear path to economic utility is defined, then drop the product and move on. One exception: If the product can be leveraged as a customer magnet and the company can monetize elsewhere along the user journey.
Why the rush?
Companies might benefit from speeding up strategy development cycles to align them with the fast pace of AI development. This includes faster PoC implementation and AI model testing between feedback loops.
The scorching pace of AI development promises to transform not just companies, but entire industries. Customers are also rapidly taking to AI and, as a result, their expectations around speed, quality and personalization are changing. AI must feature prominently on every company's business strategy. But how should companies get to grips with it and use it to accelerate growth or enhance their service offerings? First, they need to invest substantially in reliable data, the foundation for developing and trying out multiple AI models. Then they must prioritize the models which provide quick wins or promise to propel them ahead of their competitors for the long term.
AI has grown from being a mere support function, sitting at the fringes of the organization, to technology that sits at the nerve center – a crucial component of critical functions and decision-making. This marks a dramatic shift and companies have to reorient to an AI-first model. “We believe AI-first companies will be the trailblazers of the future,” says Elias Aad, Regional Head of Digital & Entrepreneurship Practice for Roland Berger in the Middle East. “They use AI to transform the wealth of data they have into smart insights which then transform their business models and value propositions to customers.”
But not all companies possess this wealth of data. Some organizations are still lagging behind due to a lack of data, or stricter frameworks around data handling and usage in some industries. Data is a strategic business asset and organizations need to dedicate adequate resources to obtaining it – or they risk competitors getting ahead of them with new, innovative products and services.
Five steps to establishing an AI-first company
- Conduct a data inventory – existing data, deficits, sources and suppliers
- Assess the organization’s AI-readiness – evaluation of data, skills and technology
- Develop an AI-first strategy – objectives and a technological roadmap
- Identify business priorities for AI leverage – quick wins and long-term gains
- Establish and execute a proof of concept – design, scope and testing
For instance, a home décor company might use AI to customize products down to the finest detail of possible customer requirements, thanks to data which consistently covers all functional and aesthetic criteria. A chatbot powered by sophisticated algorithms could support customers with their selections made online then summarize and display the order for their customized version, all within seconds. This information can feed a smart production process. Meanwhile, the AI tools can collate data and analyze it to recognize patterns across customer preferences, from most popular functionality to products which are frequently bought together. Data-driven insights like this can contribute to success, from innovative ideas for new products, to how they market their products and even which suppliers they engage with in future to meet customer expectations.
Investing in data equates to investing in AI. But what more should a company do? The following steps will help a company progress with AI development and AI-supported solutions:
Who are your AI champions?
Who is responsible for data collection, storage and management in your organization? Who will conduct inventories and evaluate data derived from external partners, to ensure it meets agreed standards and is systematically labeled and organized? If no one among your current employees is skilled in data administration then upskilling, task outsourcing or recruitment of new talent are of top priority. Equally important is securing the right people to play the role of “data and AI champions” across various business units to ensure organization-wide adoption and alignment of responsible data management practices.
An organization must have an overview of the data it currently generates and processes, identify gaps and prioritize critical data elements for their organization by considering the potential business impact of bridging these gaps.
As the data will form the backbone for new, powerful AI products, it must be evaluated with a view to processing it effectively. The sources, quality and consistency should be scrutinized, as well as how it is labeled and categorized. If key data is lacking, a plan for creating, leveraging or acquiring it must be made. C-level managers must decide on strategic partnerships with data collection service providers to gain access to relevant data assets.
Regarding management of the data, Aad explains, “Already multiple challenges have been encountered among organizations in diverse sectors, from trying to adopt a one-size-fits-all approach with data management practices across multiple business units (BUs), to a lack of standards or defined data roles throughout the organization to champion a data agenda.” A lack of robust mechanisms for the evaluation and governance of data regarding privacy and ethics has also proved problematic and must be prioritized. Organizations must adhere to data protection policies, security and ethics principles, including monitoring and governance of all related processes, decision-making and output.
Focused on data: To become an AI-first company, managers must consider and approach data as a key strategic business asset.
Embracing the prospect of more advanced AI solutions is likely to be easier for companies which experienced a digital transformation in recent years, as well as those with a deeply ingrained culture of innovation. But even they need to consider employee attitudes: the development of AI tools could completely reshape team roles and functions. For successful AI-integration, employees must be willing to work with new technologies. Leaders must be tuned in to any reluctance to work with AI tools. They also need to consider the skill sets of their workers. For example, can they write generative AI prompts in English or will they require training for this? As with all major business changes, assigning an owner for the AI agenda can help steer its implementation, overcome perceived limitations and address specific requirements and concerns.
How AI-ready is your business?
Experts at Roland Berger have established the AI Readiness Radar, a systematic assessment of all AI-related capabilities of a company. It helps businesses to define evaluation parameters to be explored as valuable input for outlining a successful AI-first business strategy.
Get in touch with us to find out more
How a company is set up impacts its ability to drive and facilitate the use of AI. Aad says, “When designing the organization structure, the proximity of AI resources to data teams is an important consideration. The functional closeness of AI teams to data-centric teams has the potential to form synergies or create silos.”
Decisions must also be made regarding short-term versus long-term AI leverage. Short-term thinking would revolve around the optimal organizational structure, governance guardrails and resource profiles needed to enable successful development of AI use cases. Leaders might also consider how AI could be developed at scale, to allow for standardization and knowledge transfer across processes, technology and tools throughout the entire organization.
“An AI strategy serves as the guiding beacon for a company’s AI initiatives by ensuring fewer ‘reactive and hype-oriented initiatives’ and more ‘strategic decision-making and AI-capability building’,” says Aad.
But how does one go about adopting an AI-first approach? A company behind a popular app might look to AI to enhance or personalize the user experience or plan to use large language models for content creation. A service provider might want to implement AI tools to take on laborious, repetitive tasks or analyze vast data sets. Leaders must take a holistic look at their own company and consider AI-driven measures to support their entire workforce in their regular business operations or where AI could completely free up time and space for teams to invest in more complex, strategic or creative endeavors.
A strategic AI-first approach would consider initiatives which
- offer the most business value as top priority – leveraging AI to do more with less and transform value creation.
- impact the entire organization, not just one business unit, and ensure synergies between organizational functions.
- are convincing and feasible enough to secure buy-in and sponsorship.
AI-round view: Leaders need to consider the benefits of AI across all business areas before they prioritize efforts and investment.
Money talks louder than chatbots
What if evaluating AI products wasn’t just about accuracy and performance, but also project unit economics at scale? If no clear path to economic utility is defined, then drop the product and move on. One exception: If the product can be leveraged as a customer magnet and the company can monetize elsewhere along the user journey.
To understand where AI could support business further, companies could revisit their understanding of customer needs and critically evaluate the services and products they offer customers. Are the services still in demand? Do the problems they typically solve for customers still exist? An industry-wide look at how AI features and solutions already serve customers, and observing current customer-AI interaction, offers insights into opportunities for new value creation in the future.
Knowing where to zero in is an essential element for the success of an AI strategy. A breakdown of an organization into functions, such as HR, Finance, etc., or by industry value chain helps determine key business activities. AI use cases can then be identifed and mapped to each activity as relevant. Long-term decisions on exploring AI capabilities would consider an organization’s people, skills, processes and the technological roadmap required for implementation.
The two main clusters of AI use cases are “time-savers” and “quality improvers” with the potential for impact in these areas:
- Personalization of the customer experience
- Improved customer acquisition, retention and sales
- Creation of new products and revenue streams
- Improved system efficiency and automation
- Increased productivity of knowledge workers
- Rapid data-driven decision-making
- Reduction in product and research innovation costs
- Improved risk prediction and management
As the prioritization of AI use cases is the most critical task in devising an AI strategy, experts at Roland Berger have developed a guideline for individual businesses to establish their own priorities for AI initiatives. Criteria include all inputs, from the business context to AI focus areas, then a look at prioritization factors which can be clustered under the overarching key benefits “business value” and “ease of implementation.” Once priority use cases have been assessed, the value behind each use case would be defined by identifying value drivers, defining the revenue and cost metrics impacted, estimating the benefts of the use case, calculating implementation costs and conducting risk and scenario analyses.
Time-Saving Use Cases
- Improved system efficiency & automation
- Increased productivity of knowledge workers
- Rapid data-driven decision-making
Quality Improvement Use Cases
- Personalization of the customer experience
- Improved customer acquisition, retention & sales
- Creation of new products & revenue streams
- Reduction in product & research innovation costs
- Improved risk prediction & management
Why the rush?
Companies might benefit from speeding up strategy development cycles to align them with the fast pace of AI development. This includes faster PoC implementation and AI model testing between feedback loops.
Companies could consider adopting a two-speed approach to AI:
Quick wins Quick wins to maintain market share through speed or personalization improvements.
Examples:
- A chatbot to answer pre-established FAQs for faster response times 24/7
- The integration of predictive maintenance in production or safety systems to avoid downtime
- Product recommendations for customers based on previous purchases
Long-term game-changers to revolutionize a company’s use of AI.
Examples:
- A sophisticated chatbot for solving all issues within an application, thus replacing specialized IT-support workers
- Enterprise search capabilities which learn from consolidated past queries and responses for faster knowledge retrieval and subsequent service offerings
Once the top priority AI use case has been identified, all relevant parameters can be determined for a proof of concept (PoC). These would include the scope, development requirements, key hypotheses to validate or test the PoC and how results will be evaluated with key stakeholders. Decision gate analysis – a “go” or “no-go” decision – for a minimal viable product (MVP) must be established along with all other MVP requirements prior to the development kick-off.
Ready-made AI models are also becoming increasingly affordable. This makes purchasing them an appealing option compared to creating a customized solution from scratch. Aad explains, “To build or to buy – and which model or mix of models to buy – is best considered strategically and depends on a company’s own capabilities and synergies, time-to-market, the cost or benefit business case, among countless other factors.”
Further factors to aid decision-making point to the human aspects of AI use, such as ethical considerations within the PoC design, sufficient involvement of humans in the AI loop and the level of personalization possible. In addition, if an AI model is to be employed in a highly accurate environment, such as cancer treatment, then model responses and outcomes must be critically evaluated to ensure they are precise, with no risk of AI hallucinations – the term used for algorithmic output based on processing incorrect, incomplete or biased data.
To get ahead in a fast-paced AI-driven world, businesses must pay attention to every AI development that could impact their industry. They must actively invest in the generation or acquisition of relevant data, as well as ethical frameworks for data management and analysis. Only organizations that are fully committed to an AI-first approach will be the ultimate winners, with diverse opportunities to explore new avenues of business.
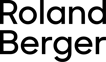